In the face of increasing environmental concerns, the construction industry is transforming sustainable and eco-friendly practices. The cities and communities heavily rely on reinforced concrete structures for various infrastructure projects due to their durability and strength. One promising avenue is the utilization of Recycled Aggregate Concrete (RAC), which minimizes the environmental impact by reducing the need for natural resources. However, accurately predicting the strength and durability of these structures, as well as detecting structural defects such as cracks, remains a significant challenge. Traditional methods often lack precision and efficiency, leading to potential safety hazards, increased maintenance costs, and shortened lifespan of concrete structures.
Artificial Intelligence (AI) and Machine Learning (ML) have far-reaching applications in the construction industry. AI has automated various facets of the construction industry ranging from progress monitoring, defect detection, automated construction progress, and many more.
This project aims to signify the use of Artificial Intelligence and Machine Learning for sustainable construction with primary focus on recycled aggregate concrete as an eco-friendly material. This project was carried out in the Military College of Engineering (MCE). Data collection involved corresponding with researchers at Guang University for access to specialized datasets, poring through Research Gate repositories and the University of California Irvine Database. The parameters of focus are the strength prediction of RAC and their performance in deleterious environments including sulfate corrosion, carbonation depth & chloride ion erosion.
Experimental investigations in the laboratory were performed initially to determine the parameters of concrete. These parameters were validated through various techniques applied in AI and ML. An optimized technique with the best results was selected to detect different crack widths and application development.
Various cracks such as normal, severe, bullet holes, and explosions were successfully detected using CNN-based models as shown in Figure 1. This part of the project will be useful in detecting various cracks at both accessible and non-accessible areas at ground and elevated structures. This aspect of the project is linked with SDG 9 (Industry Innovation and Infrastructure). This innovative technique could be used together with drone to locate and identify the severity of cracks. It will help in minimize resources, workforce and economy leading to sustainable approaches in construction which is linked with SDG 11 (Sustainable cities and Communities).
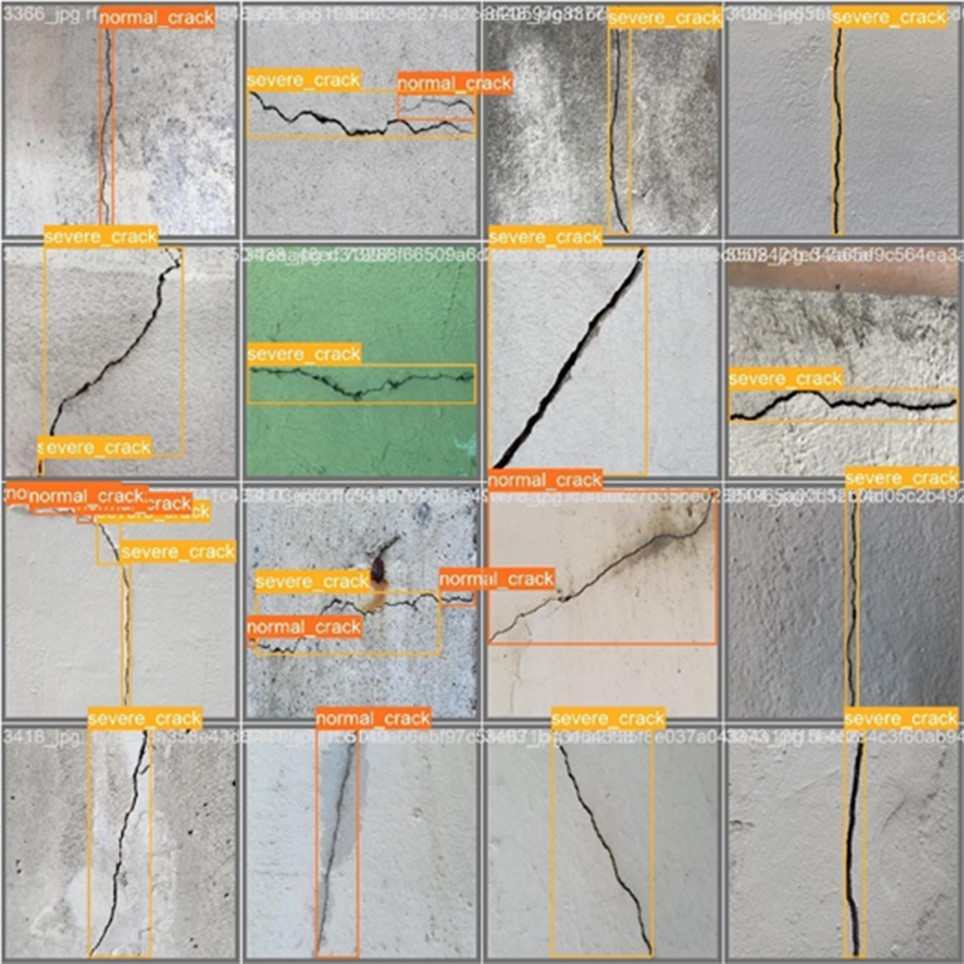
Apart from experimental work, the AI and ML techniques are applied to develop an application for predicting the strength, chloride ion, carbonation and sulphate attack of RAC. This user-friendly interface of the application was developed using Python programming script. As shown in Figure 2, the prediction of the concrete properties is based on four models that predicts the best figure with higher accuracy.
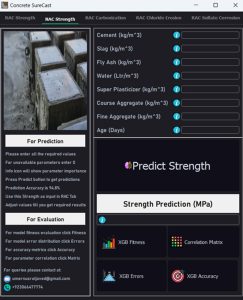
This project is unique in terms of the application of AI and ML in structures to detect various cracks. The assessment could be further studied and could be used on drones to detect cracks on long-span bridges, high-rise buildings, and places where the human eye cannot reach for study. Additionally, applying these techniques would significantly minimize labor costs, material costs and save time in carrying out lengthy experimentation.
This study will be helpful for researchers and practitioners to determine and locate structural cracks using AI and ML techniques. Additionally, the application will be helpful to predict the properties of recycled aggregate concrete avoiding going to experimentation which in turn would save time, money, and workforce.
This technique could be used commercially in structural health monitoring (SHM) of bridges, buildings, and underground structures. Together, with the industry such as contractors, consultants, and individuals, this project could be successfully implemented for business activities.
The author is Assistant Professor in Structural Engineering Department at Military College of Engineering (MCE), National University of Sciences and Technology (NUST). He can be reached at mnakhan@mce.nust.edu.pk.
Research Profile: https://bit.ly/3zvEP3p
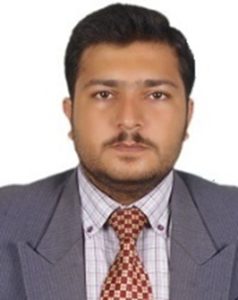