
Skin diseases are among the most common human illnesses, affecting millions of people worldwide. The World Health Organization (WHO) estimates that skin conditions account for about 1.79% of the global disease burden. Early detection of skin diseases, particularly malignant conditions like melanoma, significantly improves prognosis and treatment outcomes. Early intervention can prevent the progression of skin ailments, reduce the severity of symptoms, and minimize the risk of complications. Whole Slide Images are widely being used for skin disease diagnosis and analysis. However, prior to diagnosis the acquired specimen is processed through chemical staining to enhance the structural details.
With DermaVision, we aim at revolutionizing the domain of histopathology by introducing sustainable practices that reduce chemical usage and water wastage. Traditional histopathology practices in Pakistan are resource-intensive, with an average pathology lab processing over 2,000 slides each month. Preparing a single histology slide takes approximately 1 to 1.5 hours and consumes up to 2 liters of water per slide. Additionally, pathology labs spend an average of $2,000 monthly on staining chemicals alone.
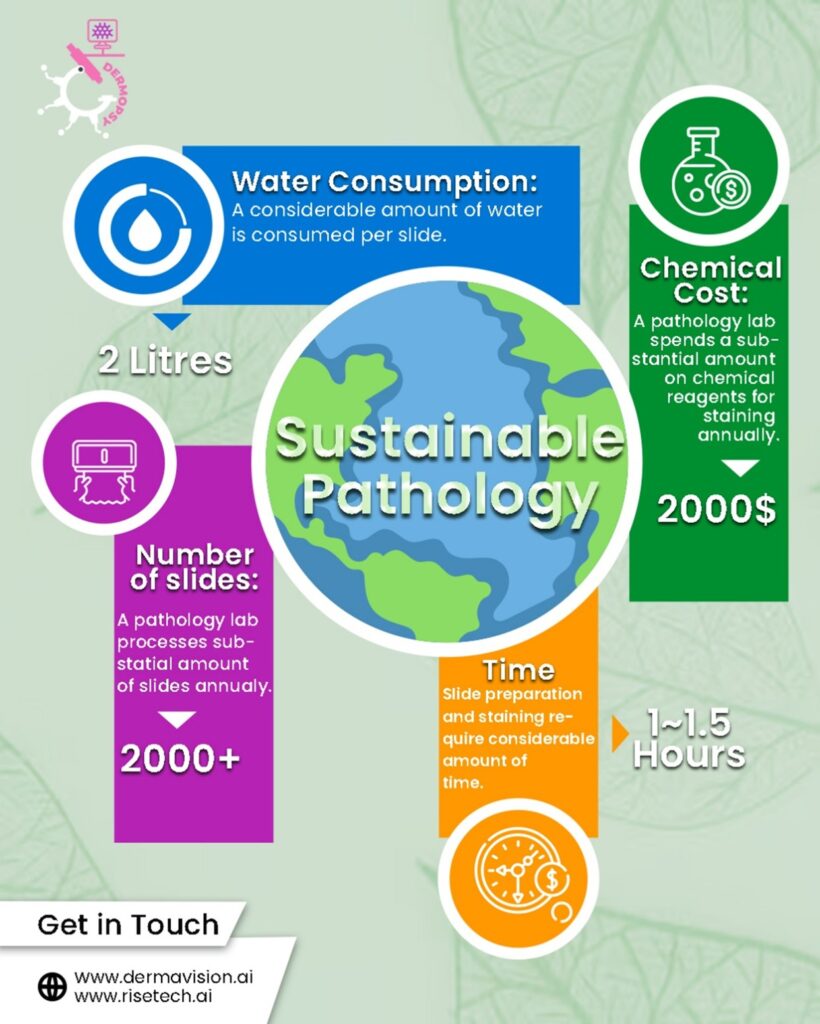
DermaVision [Figure 1 and 2] seeks to address these challenges by leveraging cutting-edge technologies to eliminate the need for chemical staining and significantly reduce water consumption, paving the way for a more efficient, cost-effective, and environmentally sustainable approach to pathology. This project has been acknowledged at various scientific national and international platforms i.e., Asia Pacific ICT Alliance Awards 2023 & 2024 at Hong Kong and Brunei Darussalam (APICTA) respectively, Pakistan Software Houses Association Awards 2023 and 2024 (P@SHA), and Prototypes for humanity at Dubai in 2024 (Prototypes for Humanity).
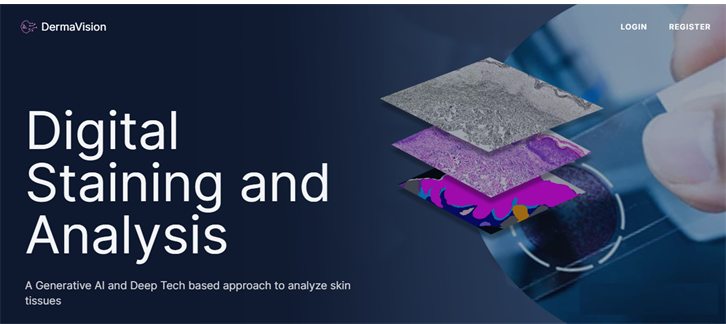
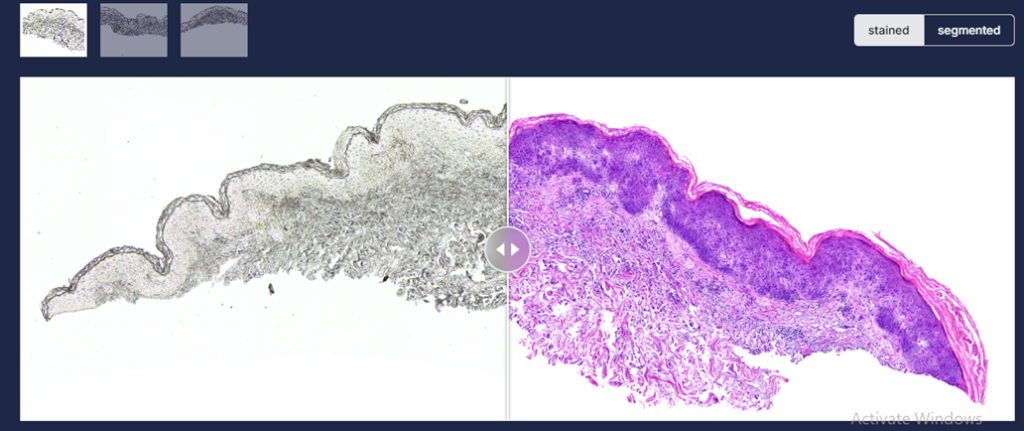
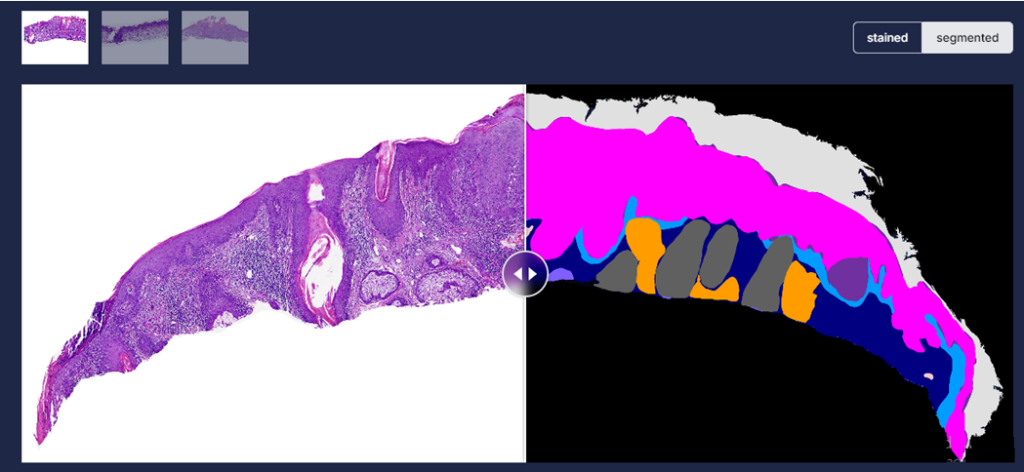
Accelerating Skin Diagnosis
Dermavision harnesses artificial intelligence to revolutionize skin pathology by analysing skin images to assist pathologists in making accurate diagnosis without extensive chemical usage or high operational costs. The framework utilized in house designed and developed generative AI models to transform an un-stained whole slide image acquired through biopsy into Haematoxylin and Eosin stained, Periodic Acid Schiff-stained and special stained whole slide images. The models [Figure 3] has been trained on proprietary data which is further made available to researchers to further grow this domain. The models have been thoroughly evaluated quantitatively and qualitatively, yielding an FID score of 80.47 and 85.17 between chemically stained (H&E and PAS) and virtually stained samples respectively.
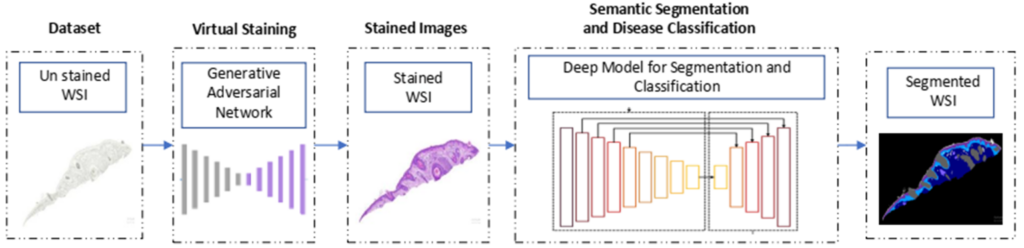
The synthesized images were also evaluated by a group of trained pathologists, for its quality of staining, resolution, image contrast and artifacts. The reviewers categorized their assessments as “Agree,” “Neither Agree nor Disagree,” or “Disagree.” The results of the majority voting-based comparison between histological and virtual whole slide imaging (WSI) of skin samples show differing levels of agreement across the five examined features. There was strong consensus in resolution (100%), sharpness (100%), and artifacts (93.3%), with dermatopathologists agreeing that virtually generated slides closely resembled histological slides. This indicates that virtual WSI successfully preserves minute details, has adequate image clarity, and effectively handles artifacts.
Image staining is followed by automated skin analysis, where a model has been trained to segment the stained image into skin layers (Dermis, Epidermis, Hypodermis, Keratin), tissue structures (Inflammation, Follicles) and skin cancer (Intraepidermal Carcinoma, Squamous cell carcinoma, Basal Cell carcinoma). Autonomous skin tissue analysis [Figure 3] has resulted in 95% over all accuracy, thus helping the pathologist identifying region of interest more accurately. Once the layers have been extracted, PAS-stained images are further utilized to localize fungus from keratin layer [Figure 4].

The project is a collaborative work between Non Invasive Diagnostic Innovations in Skin (NIDIskin), BIOMedical Image and Signal Analysis Lab (BIOMISA) and RISETech Pvt Ltd. Dr. Babar Rao a Dermoscopist and Confocalist is leading NIDISkin Team and Prof. Dr. Muhammad Usman Akram, Head of Department Computer and Software Engineering, College of Electrical and Mechanical Engineering, NUST is leading NUST team.
Publications
- Asaf, M. Z., Rao, B., Akram, M. U., Khawaja, S. G., Khan, S., Truong, T. M., … & Abbasi, M. S. (2024). Dual contrastive learning based image-to-image translation of unstained skin tissue into virtually stained H&E images. Scientific Reports, 14(1), 2335.
- Asaf, M. Z., Salam, A. A., Khan, S., Musolff, N., Akram, M. U., & Rao, B. (2024). E-Staining DermaRepo: H&E whole slide image staining dataset. Data in Brief, 57, 110997.
- Asaf, M. Z., Rasul, H., Akram, M. U., Hina, T., Rashid, T., & Shaukat, A. (2024). A Modified Deep Semantic Segmentation Model for Analysis of Whole Slide Skin Images. Scientific Reports, 14(1), 23489.
The co-author includes Ms. Anum Abdul Salam, Lecturer, at College of Electrical and Mechanical Engineering, National University of Sciences and Technology (NUST), Islamabad, Pakistan. She can be reached at anum.abdulsalam@ceme.nust.edu.pk.
Research Profile: https://bit.ly/4astb7H
The author is a Tenured Professor at the College of Electrical and Mechanical Engineering, National University of Sciences and Technology (NUST), Islamabad, Pakistan. He can be reached at usman.akram@ceme.nust.edu.pk.
Research Profile: https://bit.ly/40D78YK
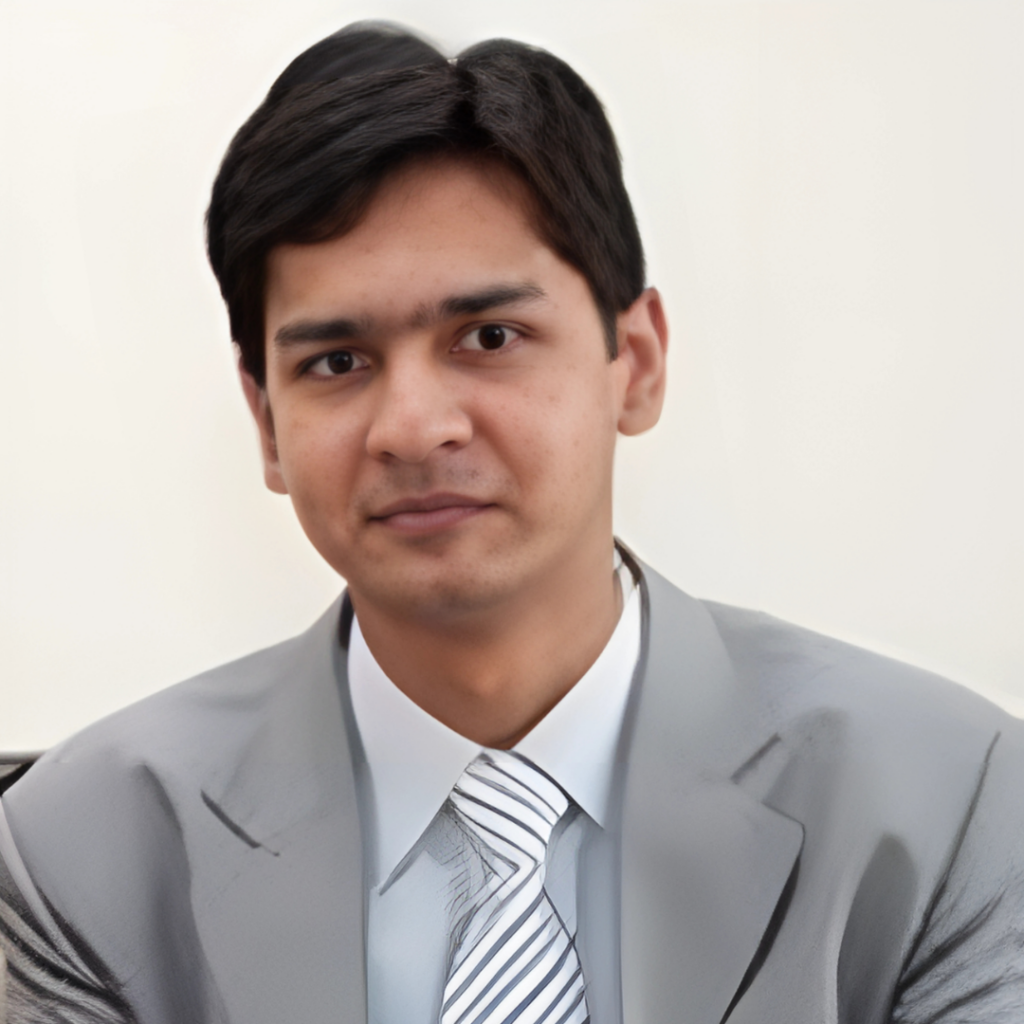