Road accidents claim millions of lives worldwide each year, making it a pressing global concern. Among the leading causes of these accidents are factors such as careless driving, drowsiness, and distracted driving. To address this issue, researchers have been actively exploring driver drowsiness detection methods. In this blog, we will discuss a recent research work [1] that proposed a state-of-the-art IoT-based Non-intrusive Automated Driver Drowsiness Monitoring Framework, specifically designed for logistics and public transport applications. This framework is aimed at enhancing road safety by effectively detecting and addressing driver drowsiness.
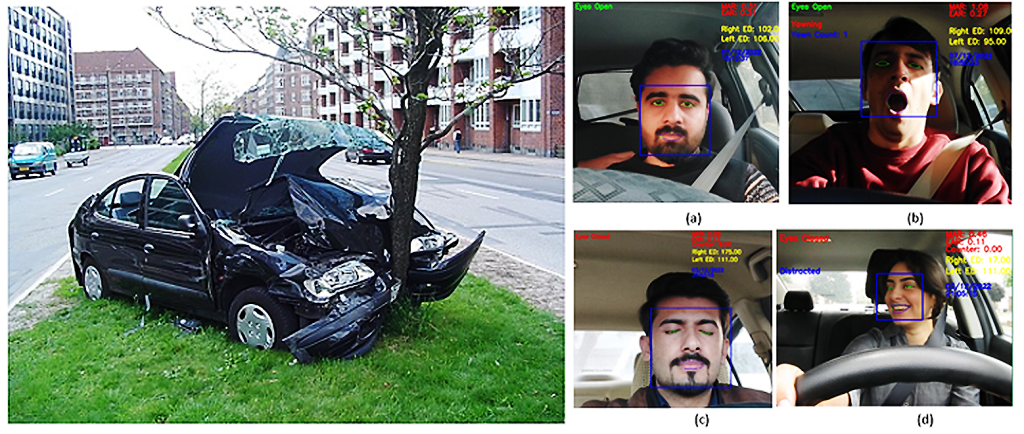
Before diving into the details of the framework, let’s shed light on the gravity of the problem. According to the World Health Organization, over 1.3 million people die annually in road accidents (Figure 1), making it the seventh leading cause of death among young people aged between 15 and 29 years [2]. Factors contributing to these fatalities include drowsiness, distracted driving, health issues, lack of road safety awareness, inconsistent law enforcement, and sleepiness [3], [4]. The statistics from the National Highway Traffic Safety Administration (NHTSA) USA revealed that, in 2017 alone, approximately 91,000 police-reported vehicle crashes involved drowsy drivers [5]. Furthermore, distracted driving claimed the lives of 3,142 people in 2020 [6]. These figures underscore the urgent need for effective measures to address driver drowsiness and its potential consequences.
The research work introduces an end-to-end non-intrusive IoT-based framework for automated driver drowsiness monitoring as depicted in Figure. 2. Specifically designed for logistics and public transport applications, this framework comprises several components working in harmony to provide a comprehensive solution. These components include an embedded system, edge computing, cloud computing modules, and a mobile phone application.
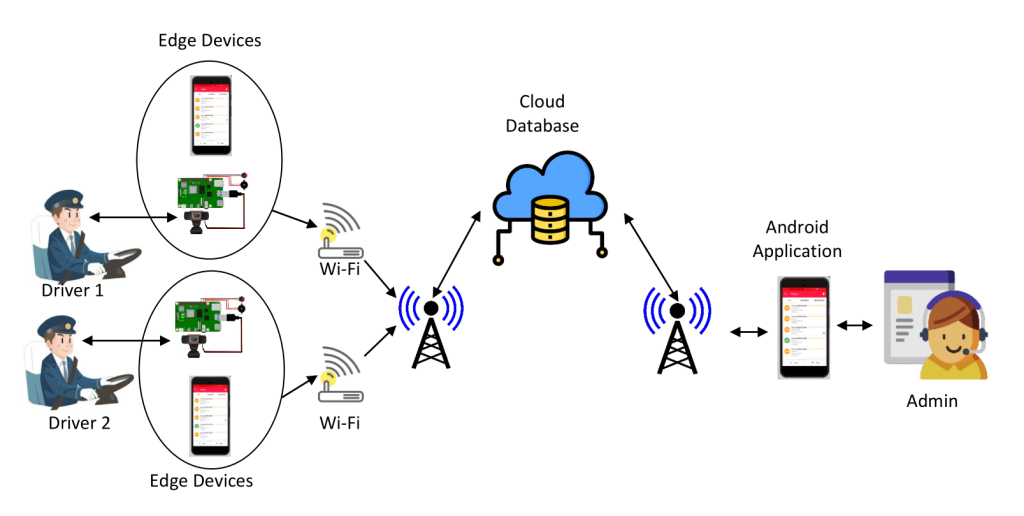
The core functionality of the framework revolves around detecting driver drowsiness and distractions using image processing techniques. By analyzing facial expressions and behavior, the system identifies signs of sleepiness, yawning, or distraction. Upon detecting any of these behaviors, the system triggers an in-vehicle alert to immediately draw the driver’s attention and prevent accidents. Simultaneously, the framework captures images of the detected behaviors along with timestamp information, current location, and route details as shown in Figure. 3. This information is stored in a cloud-hosted real-time database, which allows remote analysis of drivers’ behavior by the operators.
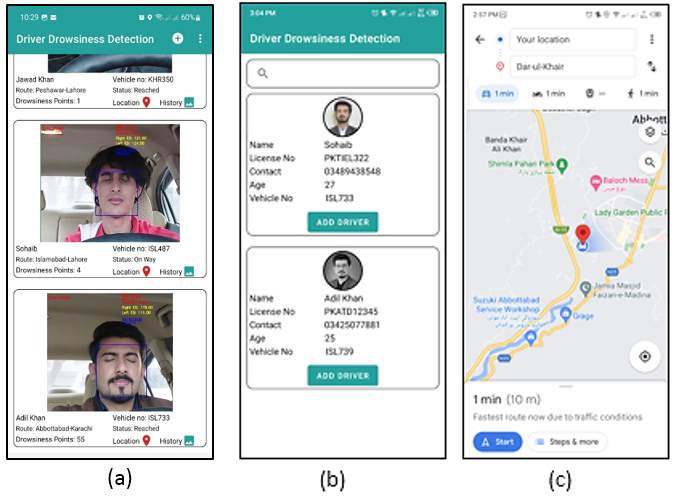
To ensure optimal safety, the framework incorporates two alert mechanisms: on-board alerts for drivers and mobile notification alerts for administrators. On-board alerts are activated when any abnormal facial actions, such as closed eyes, yawning, or distraction are detected. These alerts effectively redirect the driver’s attention, helping them avoid accidents. In more critical scenarios where a driver falls asleep (with eyes continuously closed for more than 3 seconds), the system sends an alert notification to the administrator’s mobile application. This notification includes the drowsiness images, timestamps, and location for real-time tracking.
Extensive experimental testing has demonstrated the effectiveness of the proposed framework. It achieved an encouraging overall accuracy of 96%, showcasing its robustness, portability, and usability. The framework’s ability to accurately detect and address driver drowsiness holds great promise for enhancing road safety in logistics and public transport applications.
In conclusion, the IoT-based non-intrusive automated driver drowsiness monitoring framework developed as part of this research offers an effective and efficient solution to enhance road safety based on using image processing techniques and real-time data analysis. We believe that the findings and achievements of this work could pave the way towards accomplishment of significantly reduced road accidents and increased safety for the road users.
References
[1] M. A. Khan, T. Nawaz, U. S. Khan, A. Hamza, and N. Rashid, “IoT-Based Non-Intrusive Automated Driver Drowsiness Monitoring Framework for Logistics and Public Transport Applications to Enhance Road Safety,” IEEE Access, vol. 11, pp. 14385–14397, 2023, DOI: 10.1109/ACCESS.2023.3244008.
[2] “Road traffic injuries.” https://www.who.int/news-room/fact-sheets/detail/road-traffic-Injuries (accessed Sep. 22, 2022).
[3] J. J. Rolison, “Identifying the causes of road traffic collisions: Using police officers’ expertise to improve the reporting of contributory factors data,” Accid Anal Prev, vol. 135, p. 105390, Feb. 2020, DOI: 10.1016/J.AAP.2019.105390.
[4] A. Bener, E. Yildirim, T. Özkan, and T. Lajunen, “Driver sleepiness, fatigue, careless behavior and risk of motor vehicle crash and injury: Population based case and control study,” Journal of Traffic and Transportation Engineering (English Edition), vol. 4, no. 5, pp. 496–502, Oct. 2017, DOI: 10.1016/J.JTTE.2017.07.005.
[5] “Drowsy Driving: Avoid Falling Asleep Behind the Wheel | NHTSA.” https://www.nhtsa.gov/risky-driving/drowsy-driving (accessed Sep. 22, 2022).
[6] “Distracted Driving Dangers and Statistics | NHTSA.” https://www.nhtsa.gov/risky-driving/distracted-driving (accessed Sep. 22, 2022).
The author is an Assistant Professor at the College of Electrical and Mechanical Engineering (CEME), National University of Sciences and Technology (NUST). This research was performed by M. Adil Khan (Research Associate) under the supervision of Dr. Tahir Habib Nawaz at the Robot Design and Development Lab (RDDL), National Centre of Robotics and Automation (NCRA). Dr. Tahir Habib Nawaz can be reached at [email protected].
Research Profile: https://shorturl.at/hvDQ7
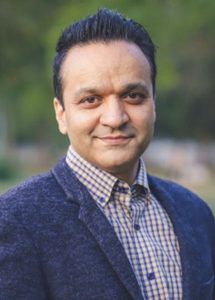