On 25 July 2000, Air France Flight 4590 took off from Paris Charles de Gaulle Airport for New York but crashed shortly afterwards taking lives of all 109 passengers on board and 04 people on the ground. The incident may have faded away in history but the repercussions of it as well as the legal battles pursued for years to come. For a common person, it may be just another aviation accident, but it gave a lot of reasons to ponder upon to those who are concerned with ensuring aviation safety. The root cause analysis eventually revealed that the fatal accident was caused due to a piece of metal strip on the runway that the aircraft ran over while taking off. That piece of metal, which was in fact a part of jet engine that had fallen from a Continental Airlines aircraft that took off few minutes prior to the Concorde, blew the tire and a large fragment of it struck a fuel tank on the underside of the wing. The spilling fuel quickly ignited, probably from an electrical arc in the landing gear wiring, and the fire caused the engines to fail.
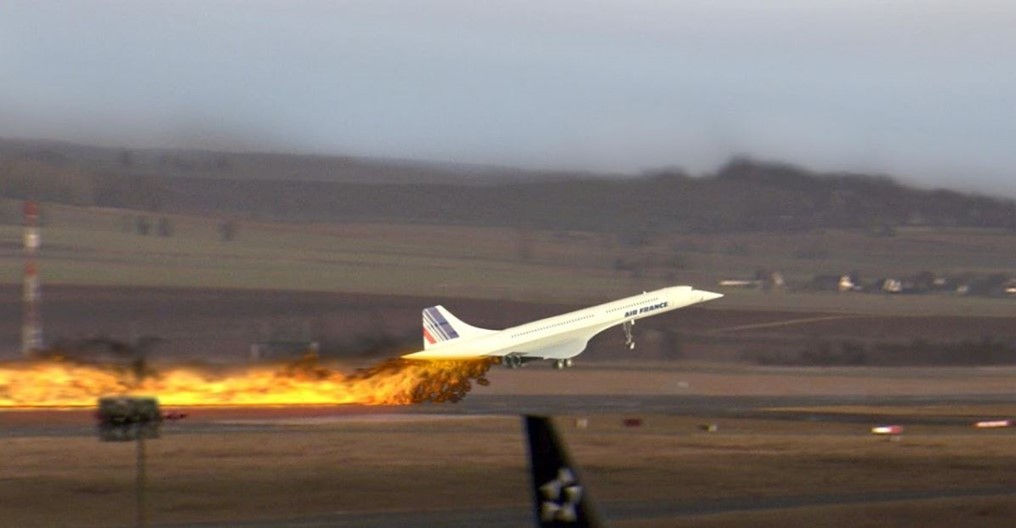
The cause of accident of Air France Flight is classified as Foreign Object Debris (FOD) or Foreign Object Damage and the incident is not the only one of its kind. Any aviation enthusiast or professional can tell you that every year FOD is known to cause considerable financial losses and damage to aircraft, both commercial and military. To curtail the incurred losses due to FOD, the aviation industry has designed procedures as well as machines to detect and rid the operating surfaces free of particles or objects that can damage the aircraft. These procedures range from manual FOD walks by airport ground staff to object detection machines that work on the principle of radars. There are also some other machines that make use of hybrid sensing principles; that is to say that results from two or more different types of sensors are amalgamated to detect and locate objects.
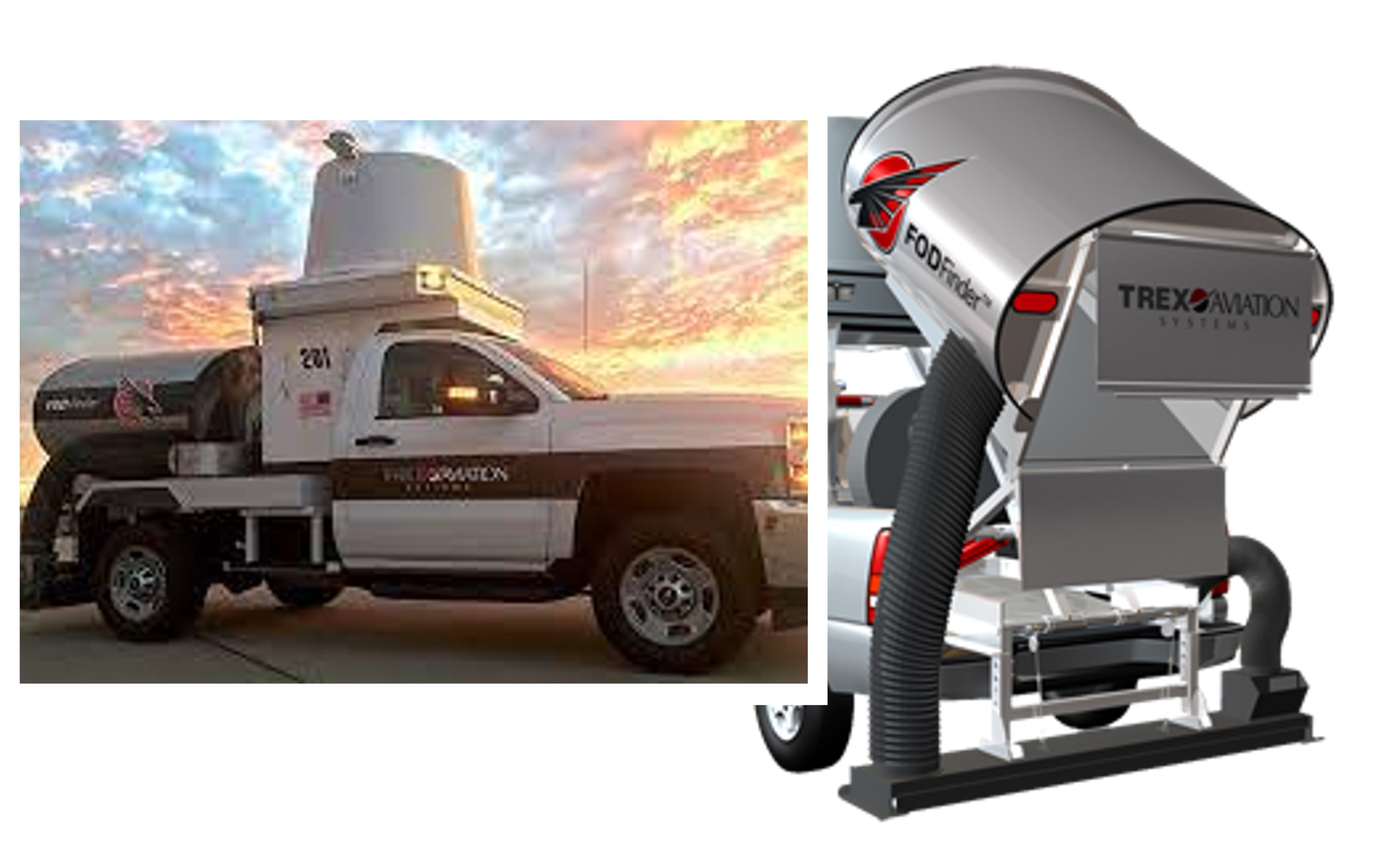
Although FOD detection systems have been around for decades, those systems are very expensive to procure; the top-end ones can cause as much as US$ 25 million or more. Therefore, in a bid to indigenously develop such systems, a team of researchers, headed by Dr. Nayyer Aafaq of College of Aeronautical Engineering (CAE), set sail on a R&D project for Automatic FOD Detection System, over a live video stream, using Deep Learning algorithm(s). For this purpose, the popular YOLOv5 computer vision model was selected as backbone architecture while FOD in Airports (FOD-A) dataset was used as a benchmark for performance comparison. YOLOv5m model is known to suffer from dataset bias that can degrade model performance for out of distribution (OOD) data. To overcome this problem, domain randomization was explored to enhance generalization of the designed FOD detection model for out of distribution (OOD) data. A novel domain randomization approach has been proposed to generate synthetic images with patch level realism to depict real-life runway predicaments like tyre marks, cracks, white-lines and taxiway yellow-lines.
After months of development and rigorous testing, the concept was eventually materialized, and a proof-of-concept prototype was put up for exhibition at IDEAS-2022 (held in Karachi from 14 – 17 November 2022) that attracted considerable attention of the audience. In a competition titled “Artificial Intelligence in Defence Market: A Paradigm Shift in Military Strategy and National Security” held at the same event it secured 3rd position, after multi-stage evaluation and rigorous scrutiny by national and international experts of the field, out of approximately 150 projects submissions countrywide.
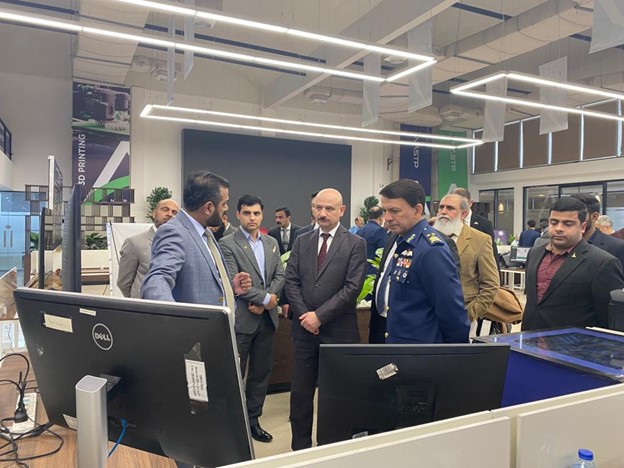
The author is MS student at College of Aeronautical Engineering (CAE), and is supervised by Dr. Nayyer Aafaq at CAE, National University of Sciences and Technology (NUST). They can be reached at [email protected] and [email protected] respectively.
Dr. Nayyer’s Research Profile: https://bit.ly/42nFAEF
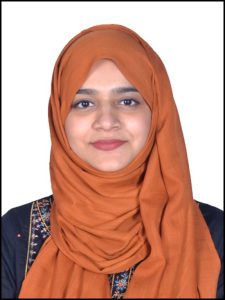