Pakistan Railways (PR), the only railroad sector organization of Pakistan aims to provide a competitive, safe, reliable, market oriented, efficient, and environment-friendly mode of passenger as well as freight transport. As of June 2022, the total track length of PR was 11,881 kilometers consisting of 445 Railway stations whereas rolling stock consisted of 466 locomotives, 1,351 coaching vehicles and 14,104 freight wagons. The passengers carried by PR increased by 26% during 2021-22 from 28.42 to 35.68 million as a result of 30% increase in passenger trains from 24,360 to 31,808 during the same period [1]. Despite these developments, the time duration from January 2021 to June 2022 was marred by 121 major accidents leading to a significant number of fatalities, injuries, and substantial damage to government and private property. The accidents were predominantly attributed to derailments, head-on collisions, fire-related incidents, engine malfunctions, and collisions with vehicular traffic at both manned and unmanned railway crossings. The safety record of PR, therefore, leaves ample room for improvement and presents an opportunity to integrate technological interventions that have the potential to avert loss of life and property while instilling confidence among travelers.
In order to address the above-mentioned safety concerns, SafeRail, a research and development project, funded by HEC’s CPEC Collaborative Research Grant (CPEC-CRG) program, is currently underway at School of Interdisciplinary Engineering & Sciences (SINES), NUST. The project has two main goals:
- To develop an Obstacle Detection and Warning System (ODWS) based on combination of optical, thermal and microwave radar technology to detect obstacles on railtracks from a far away distance and warn the drivers for in-time braking.
- To develop a track health monitoring system to identify anomalies and defects, including cracks, sleeper degradation, fishplates, fasteners, ballast quantities, track gauge, longitudinal irregularities, track geometry and horizontal alignment among others.
The complementary role of the three sensors used in SafeRail ODWS enhances the accuracy of the overall system. As detection of obstacles has to be a failsafe operation, sensor fusion approach is adopted in which each sensor’s output decision is weighed based on factors such as time of the day, environmental conditions and their individual accuracy. While optical cameras are suitable for day-time clear conditions, they cannot be relied upon in foggy, rainy and night conditions. Therefore, radar and thermal cameras, that yield better detection results in these situations, are given more weight. In this way, 24/7 and all-weather operation is ensured.
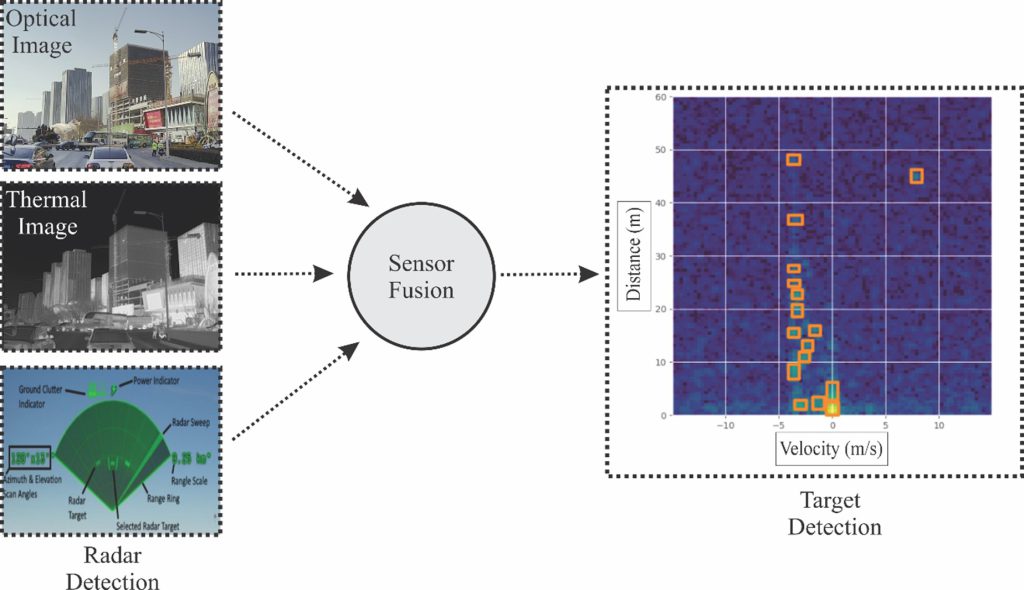
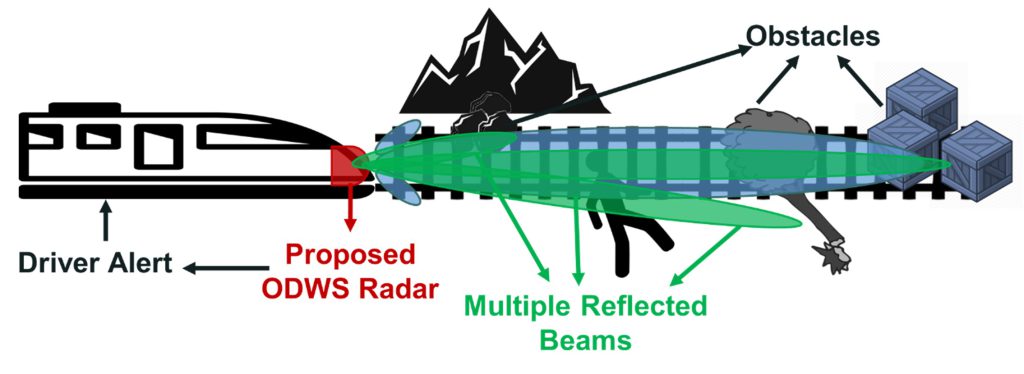
A conceptual figure showing radar’s principle of operation is shown in Figure 2. A Pulse Doppler Radar deployed in front of the locomotive will emit electromagnetic signals towards the rail track. Once these waves encounter an obstacle, they are reflected back towards the radar and based on the signature and return-time of the signal, range, velocity and target properties can be identified. The top-level architecture of Radar is shown in Figure 3 which consists of few commercial-off-the-shelf (COTS) and other custom developed components forming two major subsystems namely radar signal processing and radar antenna subsystem.
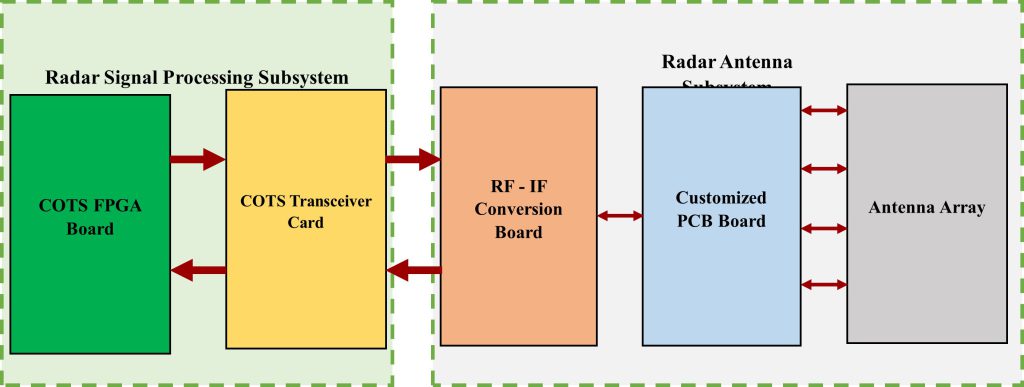
In the radar antenna subsystem, the antenna array is based on Vivaldi antennas due to their high gain, narrow beamwidth and low profile. A 1 x 4 Vivaldi Antenna Array, as a sub-set of the complete 16 x 32 is shown in Figure 4. The complete array achieves 3 deg azimuth and 6 Deg elevation beamwidth. Similarly, a 32-channel customized PCB board is also designed to provide desired input RF power to antennas. This PCB provides the flexibility of controlling the phases and amplitudes of RF signals to each channel thus enabling beam steering and tracking of targets. A four-channel subset is shown in Figure 5 which shall be replicated in eight such boards to meet the 32-channel requirement. The RF-IF conversion board down-converts the high RF frequency to baseband frequency suitable for Radar Signal Processing (RSP) algorithms on a FPGA board.
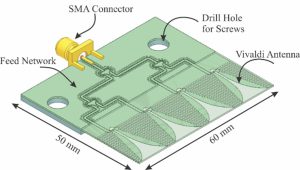
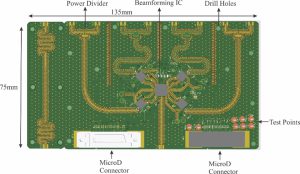
In the realm of artificial intelligence, video analytics has emerged as a powerful tool for extracting valuable insights from visual data. Smart video analytics goes beyond mere image capture; it provides capability to not only pinpoint objects within each frame but also predict their categories. To achieve this, both infrared and optical cameras are used, which together provide a comprehensive view of the dynamic environment. Deep learning algorithms are used for training on an annotated dataset that combines both thermal and optical camera feeds. During training, the model learns to map input images to their corresponding ground truth labels, which encompass bounding boxes and class categories. The algorithm achieves an optimal balance between speed and accuracy for identifying objects through YOLO-V7 model. It can detect multiple objects within a single image as shown in Fig. 6. When applied to a test image or video frame during inference, the model detects objects with F1-score of 0.959 and [email protected] : 0.973 for various classes including person, bike, vehicle and animals. The model employs the E-ELAN (extended efficient layer aggregation network) as its feature extraction backbone, significantly reducing computational and storage requirements.
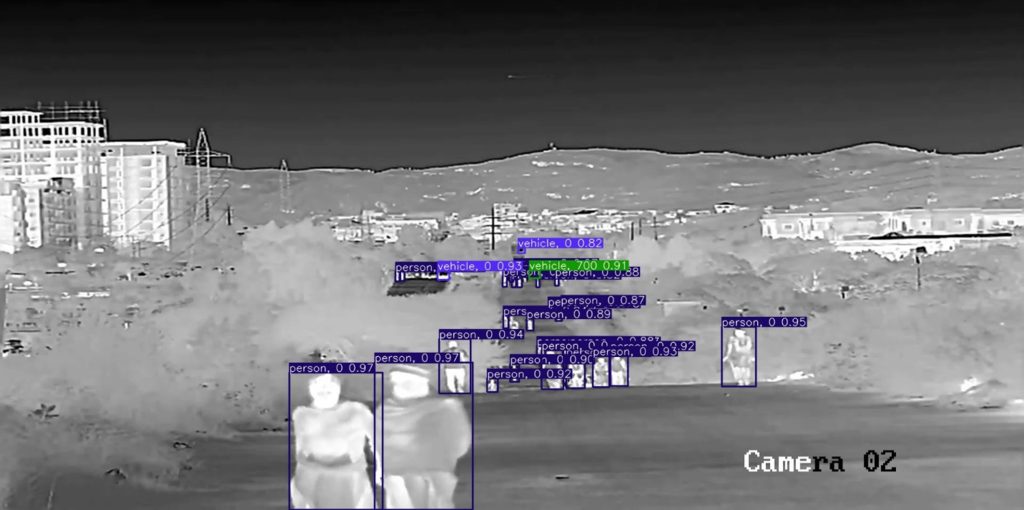
The second objective of the SafeRail project related to health monitoring of the track uses a similar image processing and AI approach to accurately detect various defects. This is achieved through collection of data using OAK-D cameras mounted on inspection vehicles. Six Thousand images, collected from Rawalpindi to Chaklala railway track, were used to train the AI algorithm and subsequently are to be used for anomaly detection in real-time. Figure 7 shows example identification for objects of interest and defects in case of a missing / faulty object.
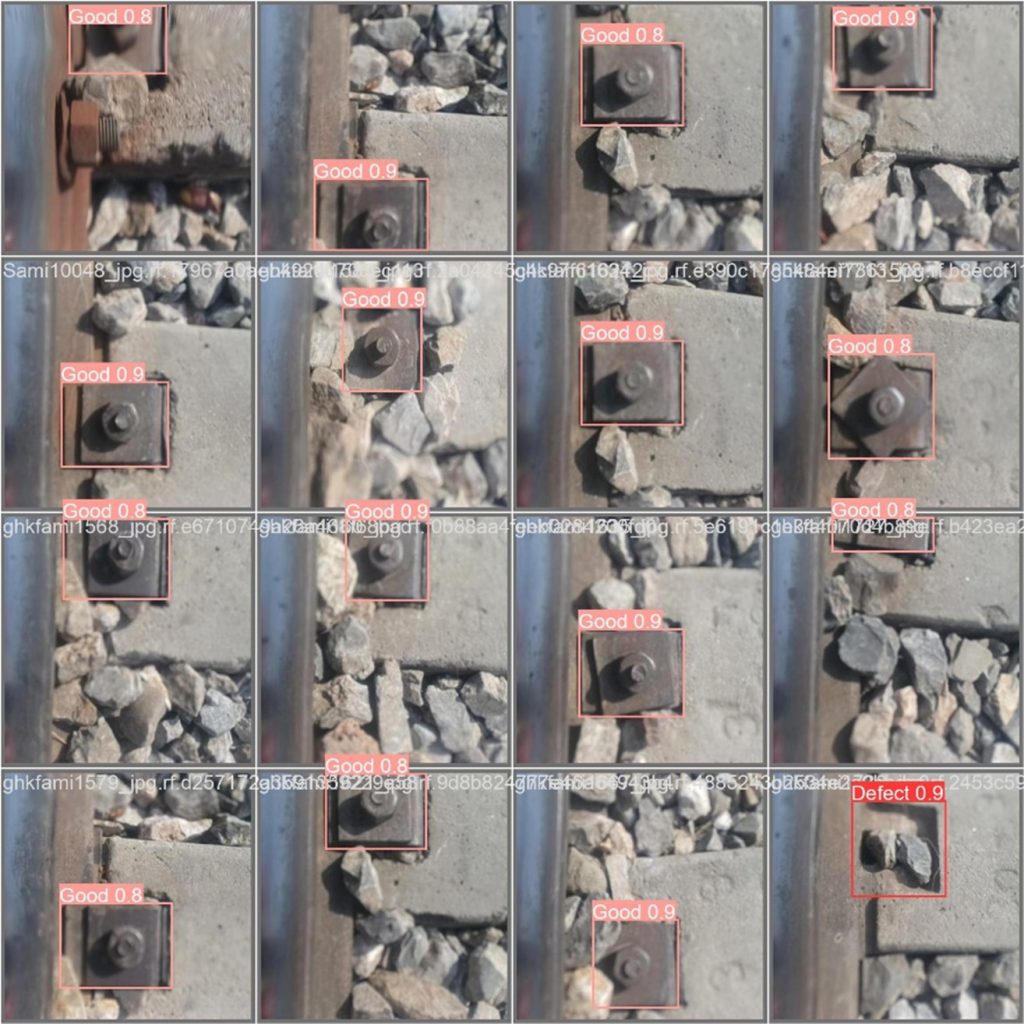
In summary, the SafeRail project is an endeavor to modernize Pakistan Railway operations and make it more safe and secure for passengers. The adopted state-of-the-art technologies and indigenous design and development, not only develops expertise in these critical areas, but also can generate multi-faceted economic activity in the long run.
References:
[1] https://www.agp.gov.pk/SiteImage/Policy/Audit%20Report%202022-23.pdf
The author is a Professor of Electrical Engineering at School of Interdisciplinary Engineering & Science (SINES), National University of Sciences and Technology (NUST) and the Principle Investigator (PI) of this project. He can be reached at [email protected].
Research Profile: https://shorturl.at/aoBSZ
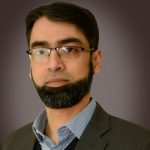